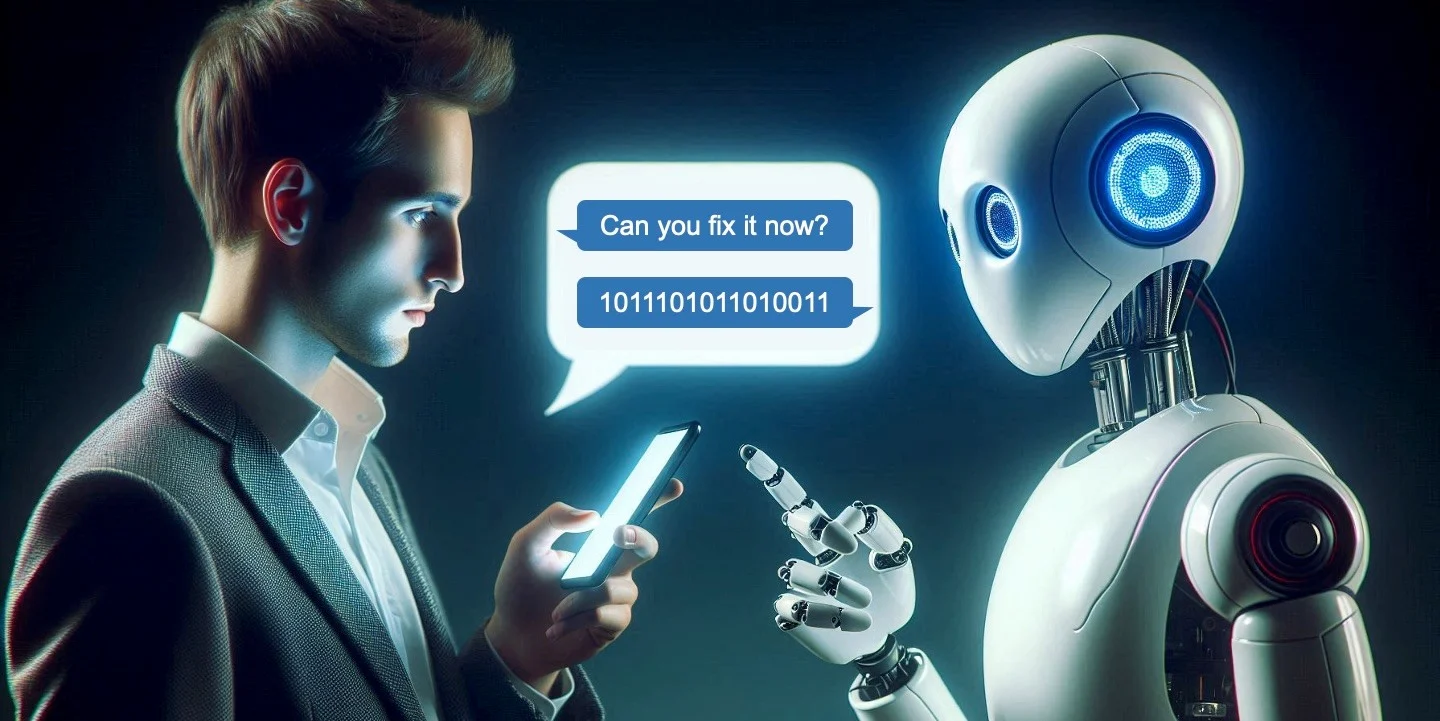
A Multi-Agent Collaborative Framework for Text-to-SQL (MAC-SQL)
MAC-SQL is an advanced multi-agent collaborative framework aimed at enhancing Text-to-SQL parsing, particularly for large databases and complex queries. It incorporates a core decomposer agent utilizing few-shot chain-of-thought reasoning, alongside auxiliary agents for refining SQL queries and managing sub-databases. Initially developed using GPT-4 to establish performance benchmarks, the framework is further enhanced by fine-tuning an open-source model, SQL-Llama, based on Code Llama 7B. With a state-of-the-art accuracy of 59.59% on the BIRD benchmark, MAC-SQL effectively addresses key challenges in Text-to-SQL tasks.
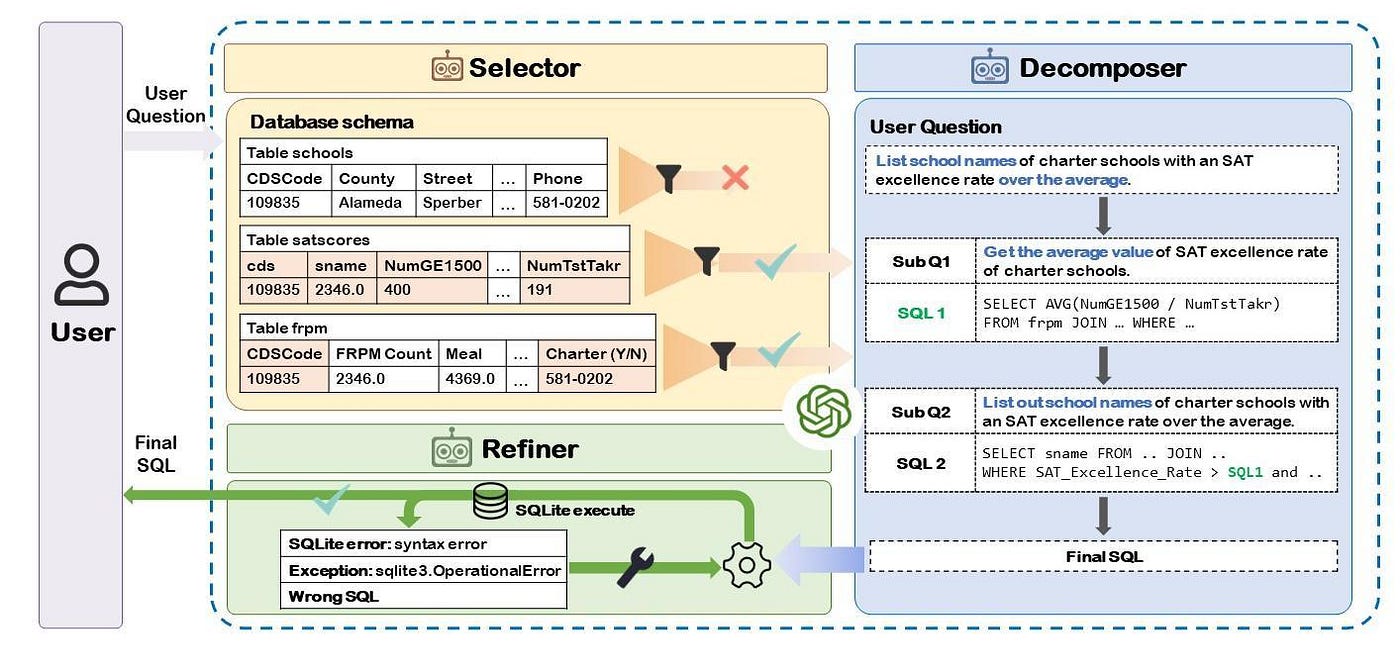
Multi-Agent Generative Approach for Text-to-SQL (MAG-SQL)
MAG-SQL introduces a multi-agent generative framework designed to improve Text-to-SQL performance, particularly on complex datasets like BIRD. Key features include soft schema linking, a targets-conditions decomposition method for handling complex queries, and iterative Sub-SQL refinement through a generator and refiner module. MAG-SQL achieves an impressive execution accuracy of 61.08% on BIRD, surpassing the performance of vanilla GPT-4 (46.35%) and MAC-SQL (57.56%).
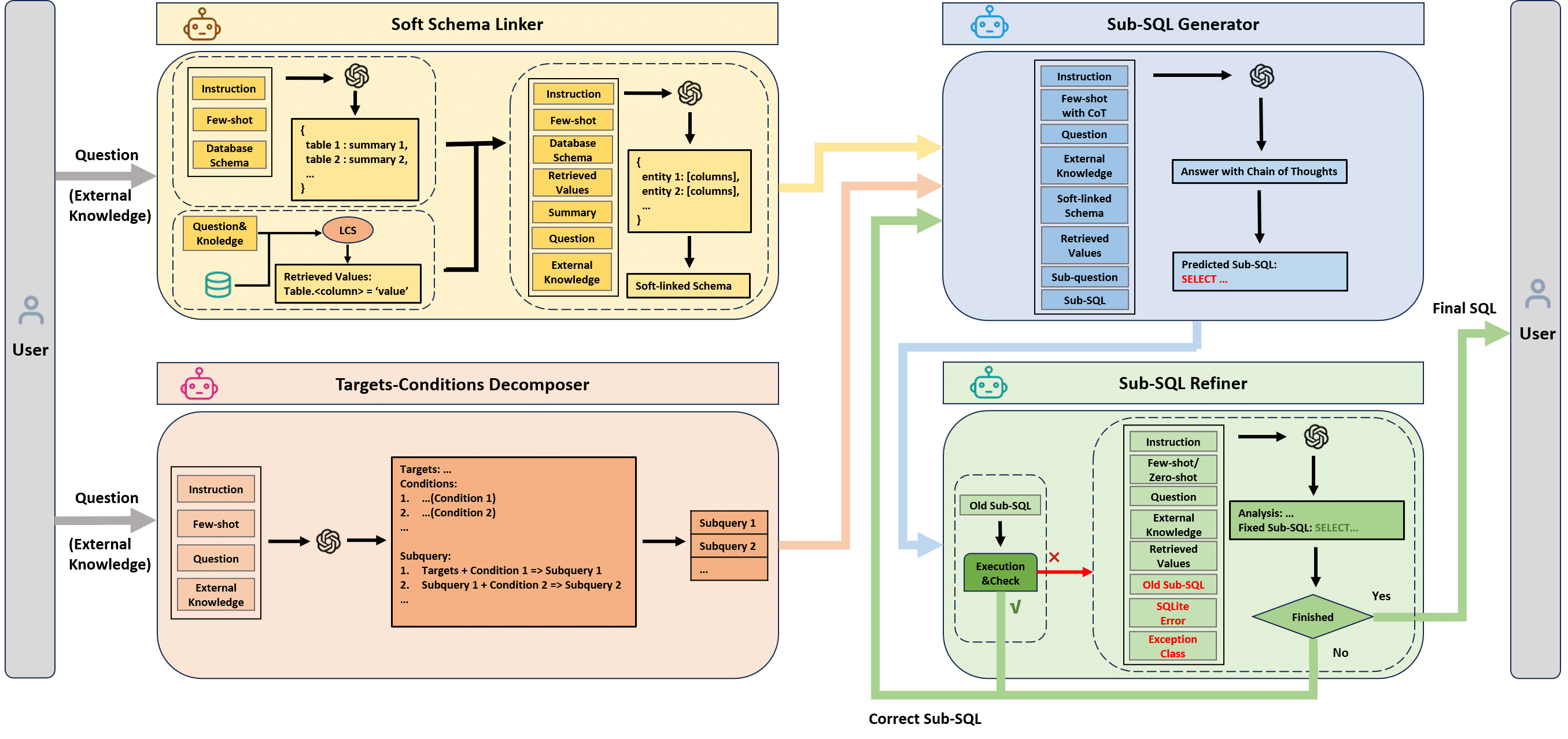
Vanna.ai: Text-to-SQL Solution
Vanna.ai is a Python-based tool designed to leverage retrieval augmentation for generating accurate SQL queries. This package utilizes large language models (LLMs) to seamlessly convert user inputs into precise SQL queries suitable for a variety of database applications.
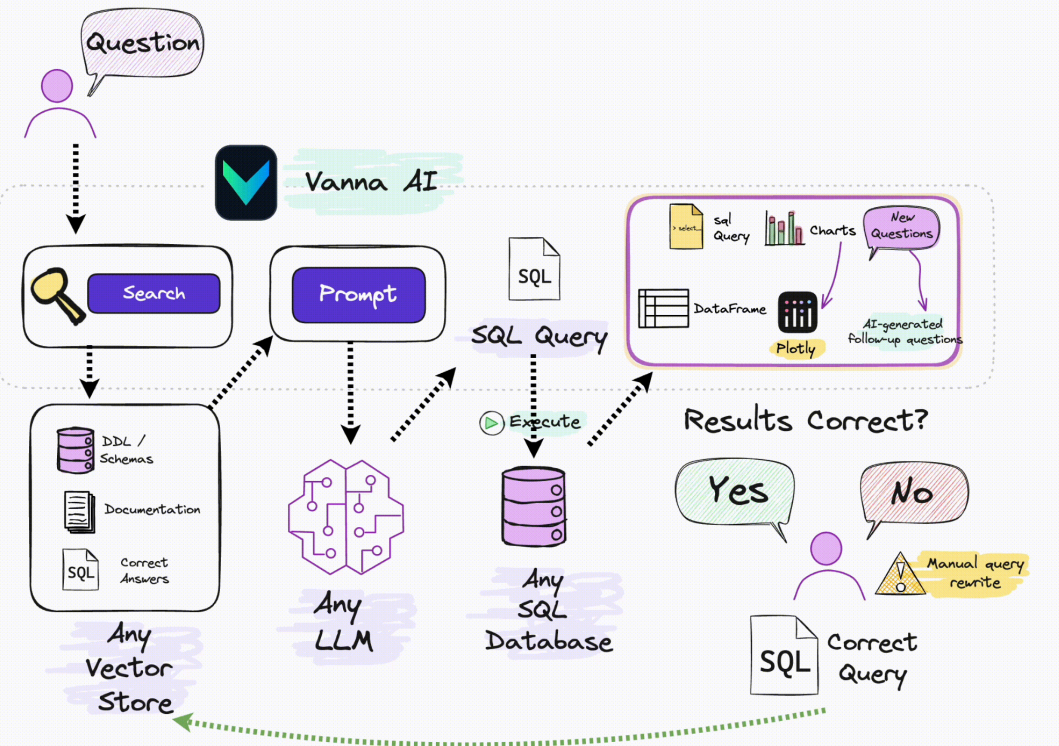